OCR vs IDP: Benefits, Comparison, & Example
This blog will help you understand OCR and IDP and how businesses can leverage these technologies to streamline daily operations. With the help of graphics, you'll see how both can be applied to improve efficiency.
Published on:
October 24, 2024
Businesses have relied on manual, labor-intensive methods to process documents for decades. Filing cabinets stuffed with paper, teams dedicated to data entry, and hours spent poring over printed forms were once the norm. This approach, while functional, was slow, error-prone, and difficult to scale. The demand for efficiency in a data-thirsty world has drastically transformed how organizations handle documents today.
Enter Optical Character Recognition (OCR) and Intelligent Document Processing (IDP) are two technological innovations that have revolutionized document management. Introduced in the early 1990s, OCR became the foundation of digitization, turning scanned images and printed documents into searchable and editable digital text. While OCR was a game-changer in its time, it was limited to basic text extraction and struggled with complex document structures.
Fast-forward to today, when IDP builds on OCR's foundation, leveraging the power of artificial intelligence (AI), machine learning (ML), and natural language processing (NLP). No longer confined to simple text extraction, IDP interprets and understands data within documents, automating entire workflows and eliminating the need for human intervention.
This blog explores the journey from OCR to IDP, how these technologies differ, and why modern businesses are shifting towards IDP to stay competitive in an increasingly data-driven world.
What is Optical Character Recognition (OCR)? The Foundation of Digitization
Well, It is the process of converting scanned images, PDFs, or photos of text into machine-readable data. OCR has been pivotal in digitizing books, bank statements, receipts, etc. Its vast applications in today’s world have made it more recognizable. Initially, when this discovery happened, only a few could use it, but now, its usability has allowed enterprises to simplify their business operations. Understanding the core, OCR translates printed characters into electronic text, making searching, storing, and editing documents easier.
How Does OCR Work?
The working principle is very simple. It starts with the scanning of documents and digitalizing with the required formats. OCR systems function by recognizing patterns in the shapes of letters and numbers. Traditionally, OCR worked by scanning documents and comparing the scanned characters to pre-set patterns stored in a database. Modern OCR technologies have evolved, leveraging machine learning to improve accuracy by "learning" from datasets to better recognize fonts, handwriting, and various languages.
.png)
Limitations of OCR
However, OCR has its limits. It struggles with complex layouts, unstructured data, and documents that require contextual understanding. For example:
- OCR may extract text from a legal contract, but it won’t understand the relationship between clauses.
- It has difficulty dealing with tables, multi-column documents, or handwritten text in various formats.
What is IDP? The Future of Document Processing
Intelligent Document Processing (IDP) takes document automation to the next level by combining AI, machine learning (ML), natural language processing (NLP), and computer vision to not only extract text but also to understand and classify it. IDP doesn’t just stop at recognition — it interprets the document’s content and context, making it a valuable tool for complex processes such as invoice processing, legal contract analysis, and insurance claims.
.png)
How Does IDP Work?
IDP operates by processing documents through multiple stages:
- Data Ingestion: Scans, emails, and other input forms are digitized.
- Classification: AI models classify documents into different types (e.g., invoices, contracts, forms).
- Data Extraction: IDP extracts relevant data from both structured and unstructured documents.
- Contextual Understanding: The AI recognizes patterns, context, and intent. It can differentiate between dates, names, and amounts and even understand complex document relationships.
Automation: Once processed, the data can automatically trigger workflows like sending emails, creating reports, or updating databases.
Key Differences Between OCR and IDP
.jpeg)
Some Real-life Applications of OCR in Action
OCR has been used in various industries for decades. Some popular examples include:
- Digitizing Books: Google Books uses OCR to scan and digitize millions of books, enabling searchable text and easier access to knowledge.
- Receipt Scanning: Apps like Expensify or Google Lens use OCR to extract text from receipts, converting them into digital expense reports.
Despite its usefulness, OCR falls short when dealing with complex documents like insurance claims or legal contracts, where structured data is interwoven with context-rich information. This is where IDP steps in.
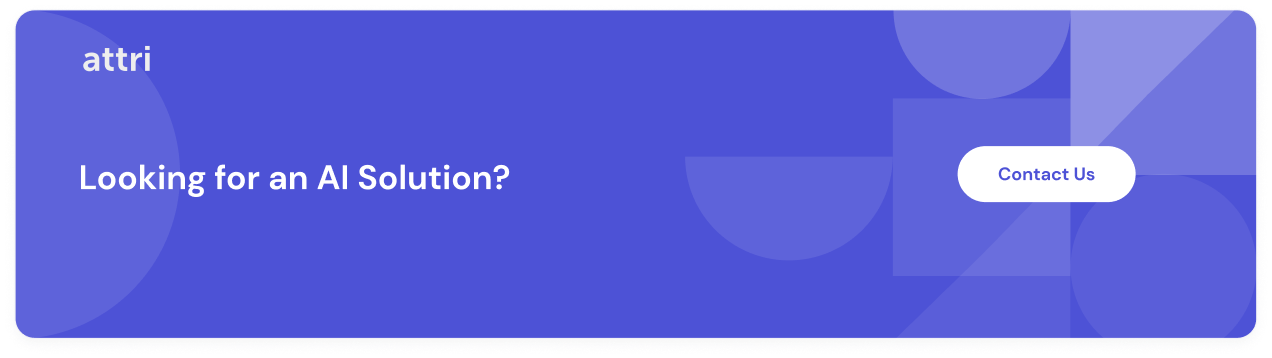
Some Real-life Applications of IDP in Action
IDP has become crucial for businesses that handle vast amounts of complex documents. Here are a few real-world applications:
- Invoice Processing in Finance: IDP can automatically scan, classify, and process invoices, extracting vendor names, amounts, and dates and then integrating this data directly into financial software. This reduces manual data entry and speeds up payment processing.
- Insurance Claims Automation: In the insurance industry, IDP processes claims forms, supporting documents, and photos. Insurers can automate approval processes by extracting and analyzing data such as policy numbers, incident details, and claim amounts, leading to faster claim settlements.
- Legal Document Analysis: IDP is highly useful in law firms, where contracts and legal agreements are common. AI models can identify key clauses, terms, and risks, automating the review process.
Why IDP Outperforms OCR
While OCR (Optical Character Recognition) has been a foundational technology for digitizing printed text, Intelligent Document Processing (IDP) takes document automation to a new level. OCR is useful for basic tasks like converting physical documents into digital formats. Still, it struggles when faced with unstructured data, complex layouts, and workflows that require more than just text extraction.
Here’s a deeper look at the key reasons IDP outperforms OCR and why businesses are embracing IDP for document processing in today’s fast-paced, data-driven environment:
1. Handling Complex and Unstructured Data
OCR is excellent at recognizing printed or typed text from simple documents, such as invoices, receipts, or books. However, its capabilities are limited when documents have complex layouts—such as forms with tables, checkboxes, handwritten notes, or multi-column structures. It often extracts the text in a raw, unorganized format, requiring manual intervention to sort and structure the data.
IDP, on the other hand, can handle complex documents with unstructured and semi-structured data. Using AI and machine learning (ML), IDP systems can:
- Interpret documents that contain tables, charts, or forms
- Extract not only the text but also understand the context and relationships between different data points (e.g., associating an invoice number with its corresponding total amount)
- Process handwritten notes, checkboxes, and other non-standard elements that would typically confuse OCR systems
By handling unstructured data efficiently, IDP dramatically reduces the manual effort required to clean and organize data, offering a much more holistic solution.
2. Advanced Data Extraction with Contextual Understanding
One of OCR's most significant limitations is that it extracts text without understanding its meaning. It cannot differentiate between text that is simply present and text that carries critical context—like dates, names, amounts, or specific terms. For example, OCR might scan an invoice and extract the numbers but fail to understand which number represents the total amount, which is a tax, or which is an invoice date.
IDP, however, goes beyond text extraction by applying Natural Language Processing (NLP) and machine learning algorithms to comprehend the data context. This allows IDP to:
- Understand different data types (dates, prices, addresses, etc.)
- Identify the relationships between entities (such as linking a policy number to a specific insurance claim)
- Extract key information and structure it in a meaningful way for further analysis or automation.
For example, IDP can process complex claims documents in the insurance industry, identify relevant policy details, and categorize various sections, like deductibles or benefits, ensuring accurate data capture for further decision-making.
3. End-to-End Automation of Workflows
While OCR requires manual review and intervention to ensure accuracy and correctness, IDP automates the entire document workflow. With OCR, you might still need human operators to verify the accuracy of the extracted data, format it properly, and feed it into other systems.
IDP goes beyond just recognizing text:
- It can classify documents (e.g., identifying whether a document is an invoice, a legal contract, or a medical record) automatically
- Extracts relevant data fields and validates the information using predefined rules (such as matching invoice amounts to purchase orders)
- Triggers downstream actions automatically, such as updating financial records, initiating payments, or sending notifications
This ability to automate end-to-end processes reduces the need for human involvement, speeds up workflows, and increases overall efficiency, saving businesses time and resources.
4. Improved Accuracy and Reduced Errors
One of the common challenges of OCR technology is accuracy, especially when processing documents with poor-quality scans, complex formats, or different languages. OCR is prone to errors like misinterpreting letters or symbols, especially when dealing with fonts that aren’t part of its training set or when documents are slightly blurry.
IDP, however, leverages AI and machine learning to improve its accuracy over time continuously:
- Learning from mistakes: IDP systems can correct and learn from errors as they process more documents. This leads to better accuracy rates, particularly when dealing with documents with inconsistent formatting or poor image quality.
- Multi-language support: Many IDP platforms are trained to handle multiple languages, handwriting styles, and document types, making them far more versatile than traditional OCR solutions.
- Contextual validation: IDP can cross-check extracted data with existing databases or rules to ensure the information is correct (e.g., validating that a detected address is legitimate by referencing a database of postal codes).
This results in significantly fewer errors, reducing the need for manual validation, improving compliance, and enhancing customer satisfaction.
5. Scalability for Growing Business Needs
OCR is primarily a tool for basic text extraction, making it suitable for smaller-scale applications or one-off document processing tasks. However, when businesses grow and need to scale their document workflows, OCR solutions often struggle to keep up, requiring more manual oversight and additional resources.
In contrast, IDP is built to scale effortlessly:
- It can easily handle large volumes of documents, processing thousands or even millions of records in real-time while maintaining high accuracy and efficiency.
- Businesses that need to process large sets of diverse document types, such as financial institutions or healthcare providers, can rely on IDP to meet their growing document automation demands without sacrificing quality.
By scaling with business needs, IDP supports future growth and ensures that companies can maintain operational efficiency as their document processing requirements expand.
6. AI-Driven Insights and Continuous Learning
OCR is static—it performs the same task repeatedly with little opportunity for improvement. On the other hand, IDP is dynamic and continually evolving as it processes more documents. AI and machine learning enable IDP systems to learn and adapt:
- Training models: IDP systems can be trained on specific document types, industries, or use cases to improve accuracy and tailor their processing capabilities to unique business needs.
- Feedback loops: With each document processed, IDP systems refine their understanding, improving accuracy and efficiency over time. This is particularly valuable in industries like legal, finance, and insurance, where document complexity varies widely.
Additionally, IDP can provide actionable insights based on the data it processes. For example, an insurance company using IDP for claims processing might gain insights into patterns related to common claim types, fraud detection trends, or operational bottlenecks—information that OCR alone wouldn’t be able to provide.
Explore Attri Resources
When to Use OCR and When to Use IDP
Understanding the differences between these two technologies helps businesses decide which tool to use based on their needs:
- When to Use OCR: OCR is suitable for simple, one-off tasks like digitizing printed materials, where text extraction is the primary goal, such as converting a printed book into a searchable PDF.
- When to Use IDP: IDP shines in processes that require intelligent automation. It’s perfect for organizations looking to digitize and streamline workflows involving complex, multi-layered documents such as contracts, invoices, or forms.
Conclusion: The Rise of IDP
OCR has played a vital role in document digitization, but its limitations are becoming increasingly apparent as business needs evolve. IDP, with its AI-driven approach, offers the ability to recognize text and understand and act on it. IDP is the clear choice for businesses looking to improve efficiency, reduce manual labor, and leverage AI for smarter decisions.
As we move into a future where automation and AI are key drivers of business innovation, the transition from OCR to IDP is not only inevitable but essential for staying competitive in a data-driven world.
Explore Our IDP Solution
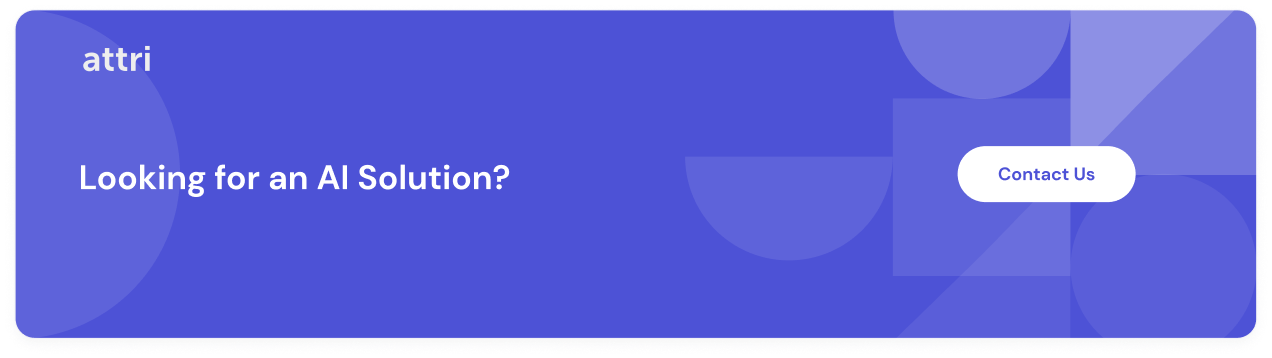