MLOps for the Modern CEO - A Detailed Analysis
Modern CEOs use MLOps to drive growth, foster collaboration, ensure data privacy/security, automate dev/deployment, and scale models. Need infrastructure/expertise/tools for successful implementation.
Published on:
October 9, 2024
Artificial Intelligence has come a long way since its inception, from simple rule-based systems to sophisticated machine-learning models that can process vast amounts of data with astonishing accuracy. AI has become an integral part of modern businesses. From automating processes to enhancing customer experiences, AI is transforming the business landscape and creating new opportunities for growth and innovation.
As a CEO, you're tasked with ensuring your company stays ahead of the curve and competitive in the ever-evolving technological landscape. Enter MLOps – the secret weapon of new-age business leaders. MLOps combines the best software engineering and data science to streamline the ML model development and deployment process, making it a crucial tool for modern CEOs who want to stay ahead of the curve. In this blog, we'll explore how MLOps is revolutionizing the business world and how it makes the modern CEO's life a tad bit simpler.
According to a survey by Accenture, over 60% of organizations are planning to adopt AI and MLOps in the next two years. (Source: Accenture)
Amazon and Unilever are two global companies that have harnessed the power of AI to improve their operations. For example, Amazon uses AI to optimize its supply chain, while Unilever uses it to develop new products. In both cases, MLOps play a critical role in ensuring that the AI models are deployed, monitored, and optimized. By leveraging MLOps, these CEOs can stay ahead of the competition and drive growth. One of the most prominent examples of MLOps in action this year is ChatGPT, a language-based AI model developed by OpenAI.
Modern CEO: A Progressive Relationship with MLOps
According to a study by Gartner, organizations that adopt MLOps can reduce their time to market by up to 30%. (Source: Gartner)
It is not a cakewalk when it comes to owning and running a business when the nature of the market keeps changing at an ever-increasing pace. Deploying and managing ML models at scale can be challenging, especially for modern CEOs already overwhelmed with a growing list of responsibilities. However, there are several ways you can use MLOps to stay ahead in your game:
- Fosters better collaboration between data scientists, software engineers, and other stakeholders in the ML pipeline. This can help your business make better use of its data and create better models in a shorter period.
- Provides a clear and comprehensive view of the entire ML pipeline, from data collection to deployment. This increased visibility can help your business understand its models' performance and potential impact, allowing for more informed decision-making.
- Provides the necessary tools and protocols to ensure the privacy and security of sensitive data used in ML models. This ensures the avoidance of costly data breaches and protects your reputation.
- Helps automate the entire ML model development and deployment process, reducing the time and effort required to launch new models into production. This results in faster and more efficient model deployment, which can lead to a competitive advantage.
- Helps the business scale its ML model to meet the demands of its growing customer base. By automating the deployment process, your business can ensure that its model is always up-to-date and functioning optimally.
- Model monitoring using ML helps you stay ahead by providing real-time insights into model performance, enabling prompt detection and resolution of issues, and improving decision-making through data-driven insights.

Success vs. Failure: Impact of MLOps Implementation
87% of data science projects never make it into production. (Source: VentureBeat)
There can be several reasons why companies fail to incorporate MLOps successfully. One reason could be the need for proper infrastructure resources to support the ML lifecycle, which can take over a year to build. Another reason could be more tools and processes to retrain production models on new data. Large-scale companies, like Netflix or Walmart, have the resources and the data to be able to afford investments in infrastructure and the hiring of additional professionals. However, most companies need more help.
Furthermore, some companies may need more expertise or resources to ensure model governance and security, which can lead to ethical and regulatory issues. Additionally, suppose a company's ML platform does not support multiple machine learning model formats or offer deployment freedom. In that case, it can limit the organization's ability to choose the best models for their needs and effectively use them in production. Finally, some companies may need visual tools for DevOps, Ops, and MLOps professionals, making monitoring models challenging and identifying performance issues challenging.
In 2021, the National Highway Traffic Safety Administration(NHTSA) declined to investigate the incidents of Tesla car battery fires, deeming them as "rare events." (Source: NBC News)
This case highlights the negative impact of the need for proper MLOps infrastructure on businesses, as it raises questions about the reliability and safety of the technology. Another study by NHTSA found that Tesla's system involved 3.2 accidents per million miles driven, higher than the national average of 2.8 accidents per million miles. This raises concerns about the adequacy of testing, validation, and safety monitoring of Tesla's machine-learning models before deployment.
According to a study conducted by Neptune in January 2023, Uber uses Michelangelo. This internal ML-as-a-service platform enables end-to-end ML workflow, including data management, model deployment, prediction, and monitoring. This Platform has been instrumental in helping Uber monitor thousands of models at scale, with over 95% accuracy in model predictions. Additionally, Michelangelo includes features for auditing and conducting traceability for data and model lineage, ensuring that all models are adequately governed and audited for usage. The API tier also plays a crucial role in managing the lifecycle of models in production, with over 70% of all models in production being controlled through this tier.
Build vs. Buy: What Should CEOs Focus On?
Build vs. Buy refers to whether to build an in-house solution to manage the deployment, scaling and maintenance of machine learning models or purchase an off-the-shelf solution.
The two cases compare the benefits of using a pre-built ML platform like Michelangelo's instead of building one in-house like Tesla's. Tesla's lack of proper MLOps infrastructure led to the NHTSA finding a higher number of accidents involving Tesla's vehicles, leading to concerns about the safety and reliability of the technology. On the other hand, the study on Uber's internal ML-as-a-service platform, Michelangelo, shows that a well-designed MLOps infrastructure can significantly benefit a company.
These cases suggest that it may be more beneficial for companies to "buy" an established MLOps platform, such as Michelangelo, rather than "build" their own. Building an MLOps infrastructure from scratch can be time-consuming and resource-intensive and may provide a different level of accuracy, governance, and scalability than a pre-existing platform.
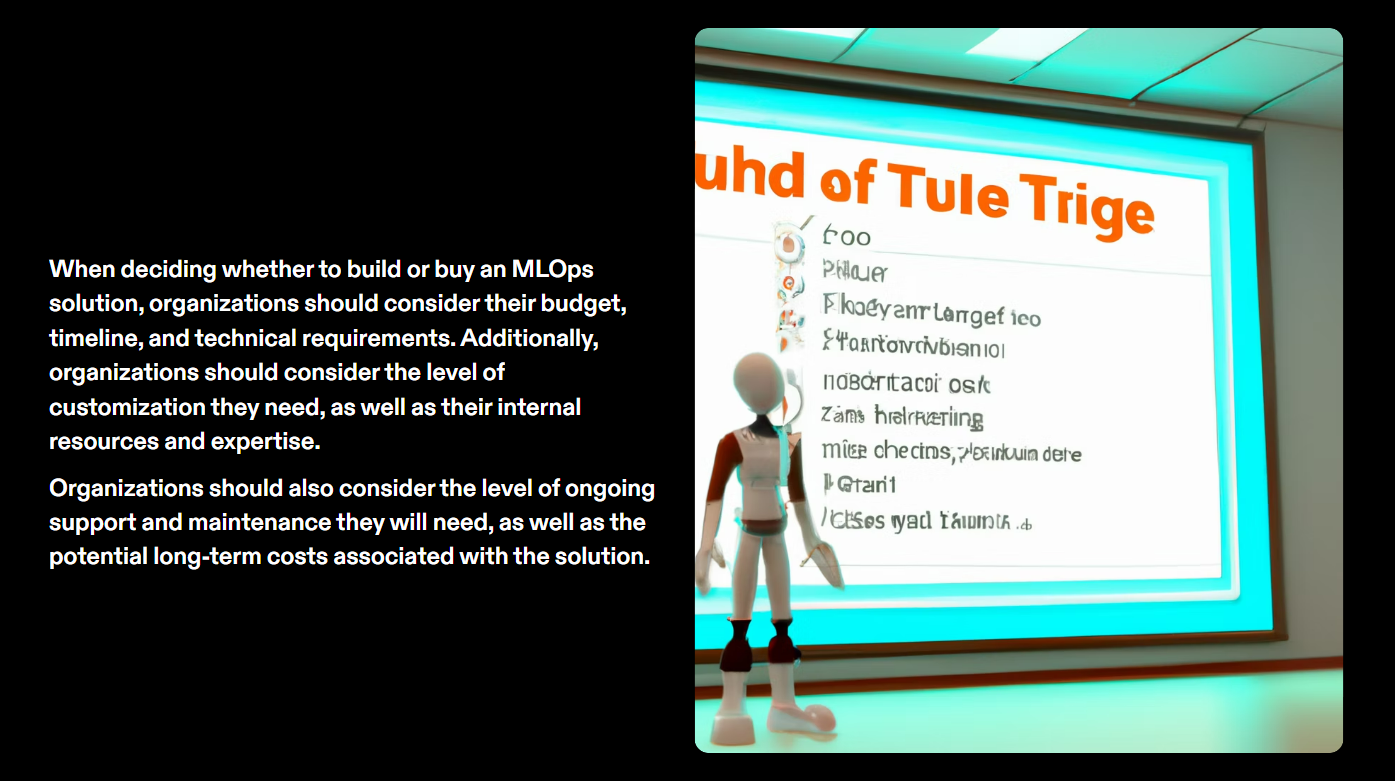
In conclusion, investing in a pre-existing MLOps platform can save a company time and resources and provide benefits such as increased accuracy and governance in model deployment and management. The "buy" option may be a more practical and cost-effective solution for companies looking to implement MLOps infrastructure.
What's Next?
As a modern CEO, you understand the importance of staying ahead of the curve and leveraging technology to drive business success. ML and AI are rapidly becoming critical components of the modern business landscape, and MLOps is the key to ensuring that your projects are executed efficiently and effectively. But with the right tools and expertise, navigating the world of MLOps can be manageable. That's where we come in.
At Attri, we understand your challenges and have the expertise to help you succeed. Our team of experts will work with you to create a customized MLOps strategy that meets your unique needs so that you can get the most out of your AI projects.
So why wait? If you want to take control of your ML projects and drive results, let us help you on your AI journey. Discover limitless possibilities with our AI Engine.