MLOps for Small to Medium Sized Businesses
SMBs are embracing AI for growth, fueling a tech revolution in sales!
Published on:
October 9, 2024
Machine learning operations (MLOps) are critical to any modern business strategy, but they can be incredibly impactful for SMBs. The adoption of MLOps among small and medium-sized businesses (SMBs) is on the rise, with these organizations recognizing the potential benefits of machine learning in optimizing their processes on a limited budget. The use of machine learning technology enables SMBs to access digital resources and save money on ICT, making it a key player in the growth of the Software as a Service(SaaS) market. With the expected growth of SaaS, SMBs can now benefit from a range of tech-led services such as digital invoicing, online transaction processing, and inventory management systems. As the tech industry continues to offer hyperlocal and specialized solutions, SMBs can use machine learning APIs to provide personalized recommendations and even assist in fraud detection. This level of personalization will not only help SMBs target their customers effectively and drive their success in an increasingly tech-led world.
Domino Data Lab introduced its newest product, Domino 5.0, the first all-inclusive machine learning operations solution designed for enterprises. This software suite is optimized to work with VMWare for running AI workloads. Businesses can now benefit from a streamlined data science process and have the freedom to choose tools that fit their needs. (Source: Yahoo Finance)
MLOps involves using data and algorithms to automate and optimize business processes, freeing up resources and increasing efficiency. The benefits of MLOps for SMBs are numerous, from increased agility and competitiveness to reduced costs and improved customer experiences. However, many SMBs struggle to implement MLOps effectively due to lacking resources and expertise. In this blog post, we will explore the fundamental principles of MLOps for SMBs and provide practical advice for businesses looking to take advantage of this exciting technology.
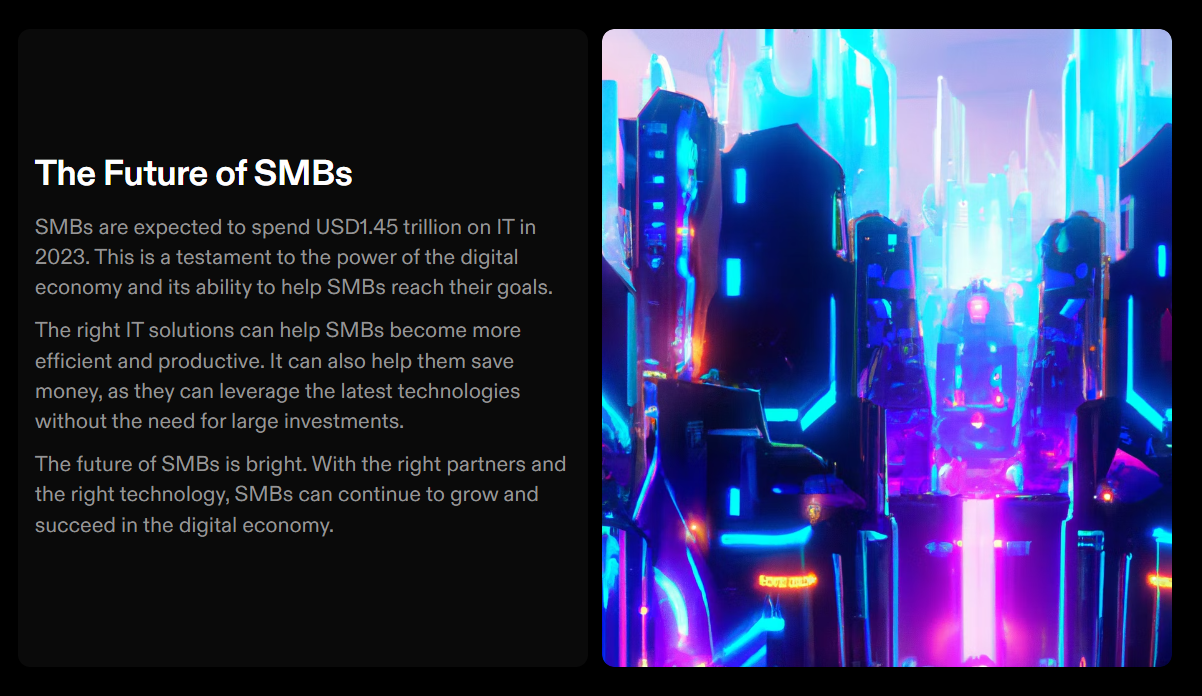
Challenges for SMBs in implementing MLOps:
- Limited Resources: Small and medium-sized businesses may need more money or workforce to employ an MLOps team or purchase expensive MLOps tools. A cost-efficient and adaptable solution is to utilize open-source MLOps platforms like TensorFlow, PyTorch, and Kubernetes. Another option is to use cloud platforms such as AWS and GCP to streamline MLOps processes and minimize the requirement for specialized MLOps staff.
- Lack of Technical Expertise: Small and medium-sized businesses frequently need help locating employees with the technical proficiency necessary to execute and oversee MLOps procedures. By collaborating with external service providers that concentrate on MLOps services, such as data scientists, machine learning engineers, and DevOps engineers, these businesses can attain the specialized technical knowledge necessary for efficient implementation and management of MLOps processes.
- Integration with Existing Systems: For small to medium businesses (SMBs) with limited IT resources, incorporating MLOps processes into their existing systems can pose a challenge. Utilizing cloud platforms and open-source tools to ease integration is advisable, which often comes with pre-existing integrations for standard tools and platforms. Another option to consider is to utilize APIs or microservices to reduce the impact on existing systems and improve integration.
- Data Management: Small and medium-sized businesses (SMBs) often need help with managing large amounts of data, especially when incorporating machine learning operations (MLOps) processes. To overcome this challenge, they can utilize cloud storage services like Amazon S3 or Google Cloud Storage to store and manage their data effectively. Data management tools such as Apache Cassandra or Apache Spark can streamline data processing and improve data quality.
- Security Concerns: Small and medium-sized businesses can encounter security difficulties when applying MLOps practices due to the absence of appropriate security measures and knowledge. Seek the assistance of professional security specialists to give direction and aid in ensuring the safety of MLOps processes. Utilize cloud-based platforms and tools with built-in security features such as encryption and control over access to secure sensitive information.
Different MLOps Solutions for SMBs and how to implement them
- Cloud-based MLOps platforms: Cloud-based MLOps platforms provide SMBs with a centralized environment to manage the entire machine learning lifecycle, from data processing and model training to deployment and monitoring. Examples of cloud-based MLOps platforms are Amazon SageMaker, Google AI Platform, and Microsoft Azure ML. Implementing these solutions involves setting up an account, uploading data, and selecting the appropriate services for each stage of the ML lifecycle.
- Open-source MLOps tools: Open-source MLOps tools provide SMBs with an alternative to cloud-based platforms. These solutions offer a flexible and cost-effective approach to MLOps and allow SMBs to host their ML models and infrastructure locally. Examples of open-source MLOps tools are Kubeflow, Apache Airflow, and MLflow. Implementing these solutions requires knowledge of cloud infrastructure, containers, and configuration management tools, such as Docker and Ansible.
- Custom MLOps solutions: Custom MLOps solutions are tailored to the specific needs and requirements of SMBs. This approach involves developing a custom platform that integrates existing tools and processes to automate the ML lifecycle. Implementing custom MLOps solutions requires in-depth knowledge of cloud infrastructure, data processing, and machine learning, as well as the ability to develop custom software.
In general, cloud-based platforms provide the easiest and most cost-effective solution for SMBs, while custom solutions provide the most flexible and tailored approach. Open-source solutions offer a balance of cost-effectiveness and flexibility but may require more technical expertise to implement.
Small Business Triumphs
GreenSteam, established in 2019, offers innovative software solutions for the maritime industry aimed at reducing fuel consumption. Excessive fuel usage incurs not only high costs but also has negative impacts on the environment. In light of this, the International Marine Organization has mandated that vessel operators decrease their CO2 emissions by 50% by 2050. Despite its relatively small size, GreenSteam has already made significant strides in machine learning with a team of 50 experts, including data scientists, developers, and domain specialists. Their products have already helped several leading shipping companies make informed decisions for performance optimization. According to Tymoteusz Wolodzko, an ML engineer at GreenSteam, the company has been at the forefront of building machine-learning products for over 13 years. (Source: Neptune.ai)
Kunal Singh, a former Uber team member, founded Discite in 2020 to help companies implement AI and ML technologies. In partnership with Eshia Solutions, Discite provides full-stack AI/ML and data solutions services to enterprises globally, focusing on computer vision, NLP, deep learning, MLOps, data engineering, and analytics. The company raised an undisclosed funding led by DynPro and is now based in Bengaluru. Discite's mission is to democratize AI for companies of all sizes and help them advance their AI strategy. For SMBs, MLOps can be a crucial tool for streamlining their operations, and Discite provides custom-made solutions to help them take advantage of this technology. (Source: YourStory)
What puts your business at risk?
- Lack of data governance: Small and medium-sized businesses often need to implement well-defined data governance rules, making their data susceptible to security breaches and poor management. To avoid this, they should develop data governance policies that specify who has access to what data and how it should be utilized. It is also crucial to educate employees on the significance of data privacy and security and implement encryption and other security technologies to safeguard sensitive data.
- Inadequate testing and validation: Small and medium-sized businesses may need to thoroughly test and validate their machine learning models, resulting in incorrect predictions and undesirable outcomes. Establishing a thorough testing and validation process, incorporating both numerical and qualitative testing, is crucial to guarantee that models are precise and align with business objectives. Simulations should be employed to evaluate how models will function in actual situations, and it may be beneficial to engage a neutral party to conduct an impartial validation.
- Underestimating the complexity of MLOps: Small and medium-sized businesses may need to fully comprehend the intricacy of MLOps, causing problems in controlling models and data. Adopt open-source tools and technologies like Kubernetes and TensorFlow to simplify the process and cut expenses. Collaborate with well-versed MLOps groups or seek advice from specialists to handle the complexities of MLOps.
- Lack of collaboration and communication: Small and medium-sized businesses may need help coordinating and communicating between various teams and departments, causing hindrances and mistakes in the MLOps process. Establishing clear communication channels and collaboration between the teams and departments is essential. Schedule regular meetings to review advancements, resolve problems, and utilize project management tools to keep track of progress and stay organized.
- Ignoring ethics and bias: Small and medium-sized businesses may overlook the moral implications of their machine learning models and disregard biases in their data, resulting in negative impacts. It is essential to educate employees on the significance of ethics in machine learning and implement fairness algorithms to reduce biases in data. Developing a code of ethics for machine learning models can ensure they are utilized responsibly and ethically.
Conclusion
The future of MLOps for Small to Medium-sized businesses (SMBs) looks promising with the increasing demand for AI in various industries. With technological advancements, SMBs can expect more affordable and user-friendly MLOps solutions that can be easily integrated with existing systems. Additionally, there will be a higher demand for specialized professionals with MLOps, cloud infrastructure, and data processing skills. This will provide SMBs with more options for collaboration and obtaining the necessary technical expertise for successful MLOps implementation.
Furthermore, there will be a rise in the development of hybrid solutions that combine cloud-based platforms and open-source tools, offering SMBs the best of both worlds - cost-effectiveness and flexibility. The increasing use of cloud computing and the Internet of Things (IoT) will also drive the growth of MLOps, as these technologies generate large amounts of data that can be used to train machine learning models.
In conclusion, MLOps is a crucial component of modern business strategies, and its importance will only increase as more SMBs adopt AI and machine learning technologies. SMBs that embrace MLOps early on will have a competitive advantage and will be well-positioned to grow and succeed in a rapidly changing technological landscape.
Ready to elevate your business to the next level with MLOps? Discover how our expert team can help you achieve a seamless and efficient ML process. Visit our website now to learn more!